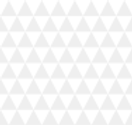
認知行動神経機構部門
Laboratory of Cognitive and Behavioral Neuroscience
Our tools
Takahashi Laboratory has developed and implemented cutting-edge research tools to investigate animal behavior and neural activity. This page provides an overview of three major tools – the Reconfigurable Maze, Neurologger, and vmTracking – including their technical details, usage in research, and relevant publications. The content is optimized for researchers, emphasizing technical specifications, data reliability, and practical considerations.
tools showcase
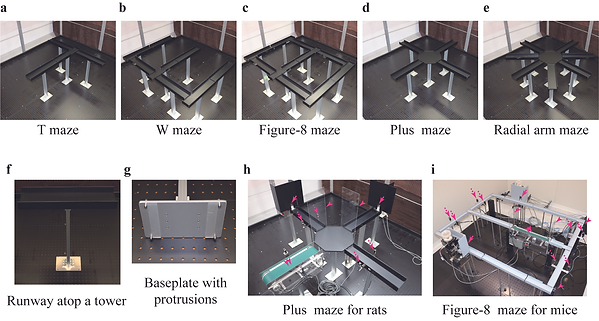
Reconfigurable Maze: Modular Behavioral Testing Platform
Overview: The Reconfigurable Maze is a flexible, modular maze system designed to overcome the limitations of traditional fixed mazes. It consists of interlocking straight and curved runway pieces and junctions that can be assembled on a grid to create different maze layouts. Researchers can rapidly rearrange the configuration to form various maze designs (e.g., linear tracks, T-mazes, radial mazes) in a repeatable and scalable manner(Hoshino, et. al, iScience, 2020). The interlocking mechanism ensures that each configuration is sturdy and geometrically precise, facilitating reproducible experiments.
Technical Details:
-
Modular Design: The maze is built from interlocking parts that snap together, allowing quick assembly and reconfiguration. Arranging pieces along a grid pattern ensures that maze geometries can be precisely replicated across trials. This design addresses flexibility and reproducibility simultaneously.
-
Scalability: By adding or removing modules, the maze’s size and complexity can be adjusted. This scalability makes it suitable for different species (e.g., mice or rats) and for testing various cognitive tasks (from simple navigation to complex memory trials).
-
Robustness: Importantly, the interlocking gaps do not disrupt animal behavior or spatial coding in the brain. Experiments showed that rodents ran and oriented normally; their hippocampal place cells were not impaired by the maze’s seams. This validates that behavioral data and neural recordings collected in the reconfigurable maze are comparable to those in traditional mazes (no artifactual effects from the structure).
-
Use and Operation: A single experimenter can assemble common maze configurations (e.g., change a rectangle to a T-shape) within minutes. The maze components are lightweight and durable (often made of plastic or resin), and the system can be cleaned or sterilized between trials. Markings or coordinates on the grid base ensure that layouts can be documented and exactly reproduced by other researchers.
Research Applications and Benefits:
-
The Reconfigurable Maze allows researchers to rapidly test multiple spatial paradigms in the same environment. For example, one can start an experiment in a simple corridor maze and then reconfigure it into a complex maze on the fly, to examine how animals adapt to changed environments.
-
Because the layout can be changed while keeping external context constant, it’s ideal for studying learning and memory. Animals can be challenged with new maze patterns without the confound of moving to a new room. This tool thus facilitates consistent investigation into the neuronal substrates of spatial learning and memory and allows efficient screening of behavioral phenotypes (Tamatsu et. al, iScience, 2024).
.
-
Proof-of-concept: In a published study (Hoshino et al., iScience, 2020), Takahashi Lab demonstrated the power of this system. They showed that smoothly “morphing” the maze’s shape (for instance, from a rectangular track into a T-maze) induces remapping of hippocampal place cells in rodents. In other words, as the animal experienced a new maze configuration, the neurons encoding its position adjusted their firing patterns, indicating the animal’s internal spatial map was updated. This finding confirmed that the maze can be used to probe neural plasticity related to spatial representations. (DOI of iScience article: 10.1016/j.isci.2019.100787)
-
Advantages: The key advantages of the Reconfigurable Maze are flexibility, scalability, repeatability, and reproducibility. A single apparatus can replace multiple fixed mazes, saving space and ensuring that results are directly comparable across different maze types. This reduces variability (since the same physical pieces form each maze) and improves throughput in behavioral experiments.
Considerations for Researchers: Researchers using the Reconfigurable Maze should ensure the modules are securely interlocked for each configuration to prevent any small gaps or instabilities. Although the design has been shown not to affect rodent behavior, it’s good practice to habituate animals to the maze and handle the pieces with gloves (to avoid transferring odors between configurations). When reporting experiments, the exact layout (and grid coordinates of walls) should be documented so that other groups can replicate the configuration. The system is patented (Japanese Patent Application P2019-115315A), and researchers interested in using it can contact Takahashi Lab for information on obtaining or constructing a similar setup.
Neurologger: Wireless Neural Activity Logger
Overview: The Neurologger is a miniaturized wireless device for recording brain activity in freely moving animals. It functions as a self-contained data logger that can be mounted on an animal (e.g., a small bird, rodent, or fish) to record electrophysiological signals (neuronal spikes, LFPs) without any tethering cables. This technology was developed to overcome the limitations of conventional neural recording systems, which typically require wires connected to head-mounted amplifiers and restrain the subject’s movement (Ide and Takahashi, Micromachines, 2023).
.
Technical Details:
-
Wireless Logging Mechanism: Traditional setups send neural signals via cables to a computer in real-time, which hampers full freedom of movement, especially in 3D space or large outdoor environments. In contrast, the neurologger digitizes and stores the neural signals locally on ultra-compact memory (such as a microSD card) mounted on the animal. No radio transmission is needed during recording, which saves power and avoids the need for continuous wireless data links in the field.
-
Hardware Architecture: A typical neurologger includes a miniature headstage with amplifiers and an analog-to-digital converter, all integrated into a lightweight package. Advances in microelectronics (MEMS technology) enabled these components to be made very small and low-power. The device usually records multiple channels of brain activity and timestamps them. Early neurologgers recorded a few channels, but modern versions can log from dozens of electrodes as memory and battery tech improve (with channel counts increasing through the 2010s).
-
Power and Data: The device runs on a tiny battery. Recording durations depend on battery capacity and memory size; some neurologgers can record continuously for hours to days. After the recording session, the device must be retrieved to download the data. The data are then synchronized with behavioral events or GPS data (if any) in post-processing. Because data are stored on-board, data integrity is high (no signal loss from telemetry dropouts), but researchers must successfully recover the device to access the recordings.
-
Attachment: Neurologgers are designed to be as light as possible (a few grams or less) to be carried by animals with minimal impact on their natural behavior. They can be affixed using a harness, backpack, or surgical mounts (for implants) depending on species. Proper attachment is crucial: it should be secure enough that the animal cannot dislodge it during movement (or in water), but also comfortable to avoid impeding the animal.
Research Applications and Benefits:
-
The neurologger enables neuroethological studies, meaning researchers can study brain activity in natural behaviors and habitats that were previously impossible to monitor. Because the animal is untethered, one can record neurons during flight, swimming, or wide-ranging exploration– scenarios where traditional setups fail. Takahashi Lab has utilized neurologgers to investigate spatial cognition in truly natural settings. For example, they have examined the neural correlates of 3D flight in birds and underwater navigation in marine animals by deploying neurologgers in those animals’ natural environments.
-
A landmark result using this technology was published by Takahashi et al. in Science Advances (2022). In that study, neurologgers were attached to juvenile streaked shearwater seabirds to record head direction (HD) cells in the brain during the birds’ first exposure to outdoor environments prior to migration. The neurologger captured neural activity as the birds oriented themselves in different directions. Remarkably, the recordings revealed that the HD cells in these birds’ brains have a preferred firing direction toward geographic north, and this preference remained consistent regardless of the bird’s location or visible cues. This finding suggests the birds possess an internal compass: a neural mechanism tuned to the Earth’s magnetic north. Such insight was only possible by recording brain activity in unrestrained, naturally behaving animals. (DOI of Science Advances article: 10.1126/sciadv.abl6848)
-
Advantages: The primary advantage of the neurologger is the ability to record high-fidelity neural data in the field or in free-moving conditions, combining behavioral and neural observations. It untethers neuroscience from the lab, allowing studies of navigation, migration, social interaction, and other complex behaviors in the wild. Data collected can reveal brain dynamics during natural tasks (e.g., long-distance navigation, predator-prey interactions) that cannot be replicated indoors. Additionally, since data are stored onboard, recordings are not limited by range; an animal can travel far from the researcher (or even out of sight, such as diving underwater or flying) and the data will still be safely recorded for later analysis
Considerations for Researchers: Using neurologgers requires planning for device recovery – for wild animals, this may involve tracking the animal via GPS or radio to retrieve the logger. The weight of the device must be a small fraction of the animal’s body weight (a common guideline is <5% of body weight) to avoid impeding normal activity. Researchers should also be mindful of the sampling rates and memory limits: high-frequency recording of many channels can fill memory quickly, so configurations often balance resolution with duration of recording. It’s important to sync the neurologger’s clock with any external sensors or video beforehand, since alignment of neural data with behavior is done after data retrieval. Lastly, ensure animal ethics and welfare: since the neurologger involves attaching a device to an animal, proper ethical approval and humane handling are required. When used carefully, neurologgers provide reliable, high-precision neural data from environments previously off-limits to neuroscience.
vmTracking: Virtual Marker Multi-Animal Tracking
Overview: vmTracking (Virtual Marker Tracking) is a software-based tool for high-accuracy pose tracking of multiple animals in video data, even in crowded or high-occlusion scenarios. It addresses a common challenge in behavioral research: when multiple animals interact, automated tracking algorithms often lose track of individual identities due to occlusions (animals overlapping or obstructing each other). vmTracking, developed by Takahashi Lab, enhances existing machine-learning tracking methods by introducing “virtual markers” to maintain consistent identities of each subject throughout the video (Azechi & Takahashi, PLoS Biology, 2025).
.
Technical Details:
-
Markerless Tracking Background: Modern pose estimation frameworks like DeepLabCut (DLC) and SLEAP can track animal body parts without physical markers, but when animals collide or cross paths, the algorithms may confuse who is who. Identity switches and dropped tracks are more frequent in occlusion-crowded scenes.
-
Virtual Markers Concept: vmTracking augments markerless tracking with a virtual labeling step. After initial detection of animals, virtual markers (unique labels) are assigned to each individual within the video frames. These are not physical tags on the animals, but rather metadata tied to their image coordinates. For example, two mice in a video might each be overlaid with distinct colored labels or IDs in the analysis software. These virtual markers serve as persistent identifiers.
-
Tracking Pipeline: Using the virtual markers as anchors, the system then applies single-animal tracking algorithms to each individual separately. In practice, this means the multi-animal video is converted into multiple streams (or an algorithmic process) where each animal is tracked by a dedicated instance of a single-animal tracker (like running DLC separately for each identified individual). Because the individuals have been “marked” in the data, the tracker knows which animal is which, preventing identity swaps even if they come into contact. The virtual markers carry through occlusions – once an animal reappears, the system recognizes it by its marker attributes.
-
Reduced Occlusion Error: By combining the strengths of markerless tracking (no physical markers needed on animals) with the identity persistence of marker-based tracking, vmTracking achieves robust multi-animal tracking in crowded environments. The developers report significantly fewer instances of lost tracks or identity errors, and a reduction in the manual corrections typically required to fix tracking data.
-
Integration and Output: vmTracking isn’t a standalone software but rather a methodology built on existing tools. It requires an initial training of a pose estimation model (e.g., a DLC model that can detect the animals) and then a procedure to assign virtual markers and run the tracking. The output is time-series data for each animal’s poses/positions, with identities reliably maintained. The method is flexible: it was implemented in both DLC and SLEAP pipelines, termed vmT-DLC and vmT-LEAP respectively in the publication, showing it can integrate with different tracking software. The code and data for vmTracking analyses have been made available via the authors (Zenodo repository) for reproducibility.
Research Applications and Benefits:
-
Behavioral Studies with Groups: vmTracking is ideal for experiments involving social interactions, group dynamics, or any scenario with multiple animals in the same arena. For instance, researchers studying mating behaviors, dominance hierarchies, or cooperative tasks in rodents can use vmTracking to get accurate movement and pose data for each individual simultaneously, even if the animals huddle or tussle.
-
Complex Environments: In more naturalistic arenas where animals have hiding spots or dense environments (e.g., enriched habitats, maze with multiple animals), occlusions are common. vmTracking helps maintain continuous data on each animal. This leads to more complete and reliable datasets for analysis of social or interactive behavior.
-
Demonstrated Success: Azechi & Takahashi (PLOS Biology, 2025) demonstrated vmTracking’s performance across different species and scenarios. They tested it on groups of mice in a cage, schools of fish in a tank, and even human dancers in a studio, all scenarios with frequent occlusion. vmTracking consistently achieved higher accuracy than traditional multi-animal tracking; it minimized the number of manual corrections and identity errors by nearly 50%, dramatically improving efficiency. The approach proved broadly applicable: from small fast-moving animals to larger slower-moving subjects, indicating its utility in diverse research fields. The authors note that this advancement can enhance the precision and reliability of behavioral data in studies of complex social systems, providing a simpler yet more effective solution for multi-animal tracking. (DOI of PLOS Biology article: 10.1371/journal.pbio.3003002)
-
Data Integration: Accurate multi-animal tracking data from vmTracking can be synchronized with other measurements, such as neural recordings (e.g., if multiple animals are each implanted with neurologgers or other devices) or physiological sensors, enabling comprehensive studies of how social interactions influence brain and behavior. It also opens possibilities in ecology (tracking animals in groups via video), and in computational biology for building better collective behavior models.
Considerations for Researchers: Implementing vmTracking requires some familiarity with pose-tracking software and data handling. Researchers should be prepared to label some video frames for training the initial markerless tracker (DLC or SLEAP), as usual for those tools. Once a baseline model is ready, adding the virtual marker step involves additional scripting or using the authors’ provided code. Ensuring good video quality (sufficient resolution and frame rate) is important – the better the animals’ features are captured, the easier it is to assign and maintain virtual markers. There may be scenarios of extreme occlusion (e.g., one animal completely hides behind another for a long duration) where even vmTracking can struggle; careful experimental design (such as using overhead cameras and maybe multiple angles) can mitigate this. The computational load of vmTracking is a bit higher than standard tracking since it may run multiple tracking processes in parallel (one per animal), so access to a GPU and time for processing should be considered for large datasets. Overall, for those with the technical setup to deploy it, vmTracking offers a high-accuracy, validated approach to multi-animal tracking that significantly improves data quality in behavioral research.
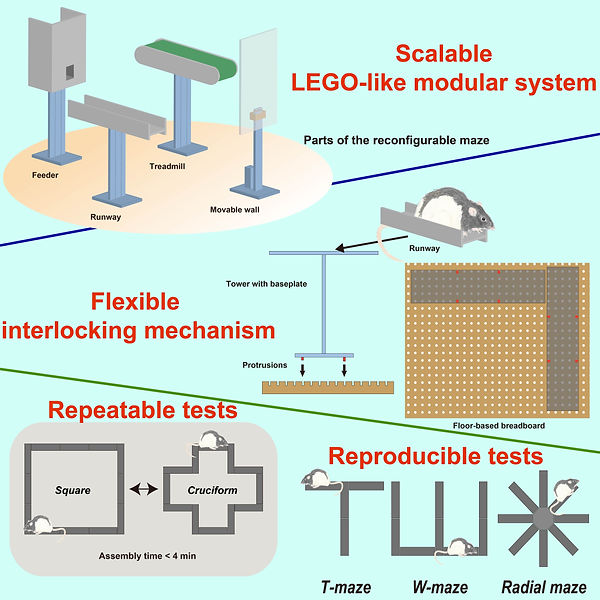
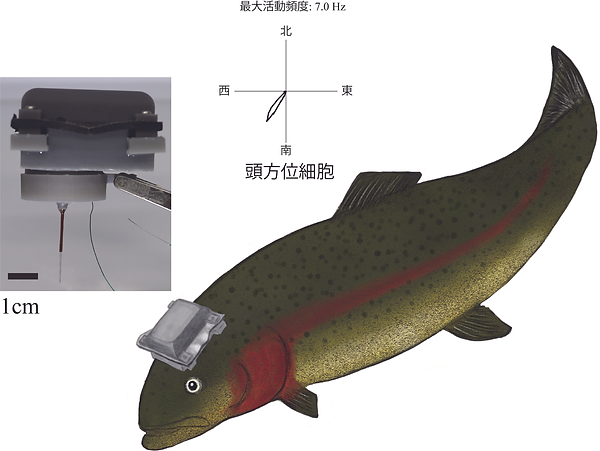